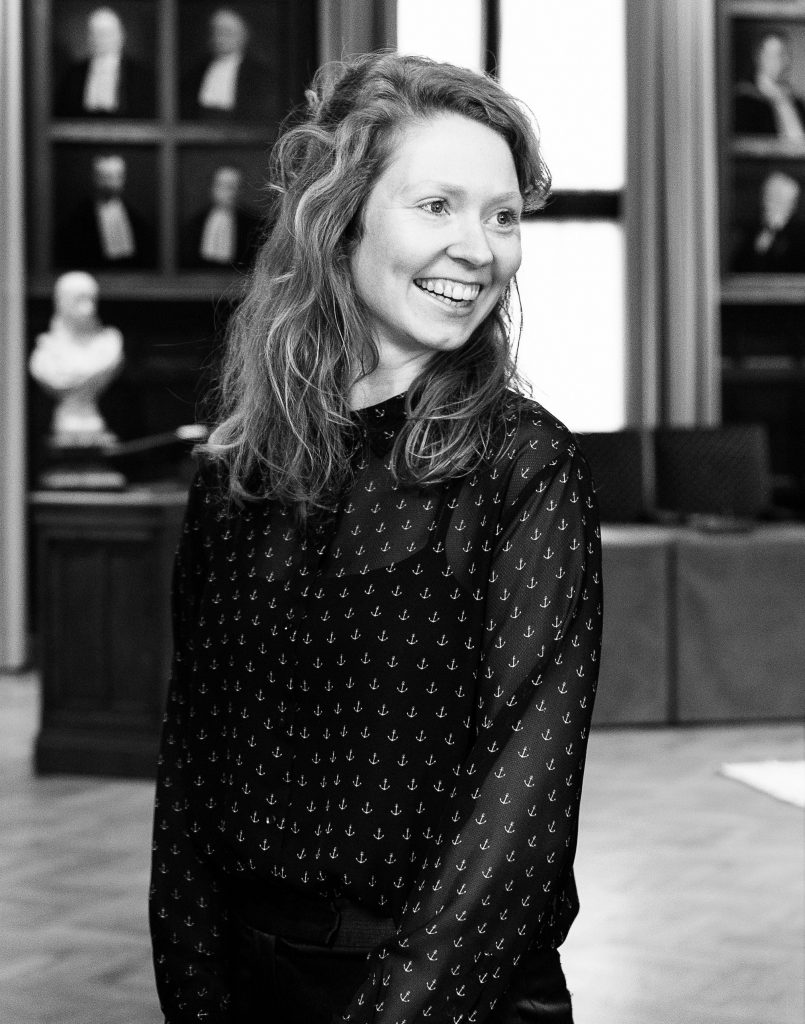
Methodology & Statistics
Faculty of Social Sciences
Utrecht University
On 2 June 2023 Sanne Smid defended her thesis Bayesian SEM with Small Samples: Precautions and Guidelines, at the Utrecht University.
Summary
Sometimes it can be challenging to collect enough data. Think of naturally small populations, such as people with rare diseases. Or hard to access target groups, such as people with addiction problems or undocumented migrants. However, all statistical methods require a certain amount of data to perform well. Recently, more and more often researchers with small samples switch to a Bayesian approach to deal with analysis problems. In the Bayesian framework, observed data is combined with prior knowledge (e.g., knowledge based on opinions of experts in the field or based on previous studies). This prior knowledge is captured in a distribution, the so-called prior distribution.
The studies in this dissertation show that the switch to a Bayesian approach is not without problems. Some software programs offer built-in default prior distributions, which are unfortunately not always suitable and can lead to incorrect results when samples are small. The use of Bayesian statistics in that case is not a solution to analyse a small sample. Blindly relying on built-in default priors is not an option when samples are small. The smaller the sample size, the more important the prior distributions.
In Chapter 1 of this thesis, the results are presented from an extensive systematic literature review on the performance of Bayesian and frequentist estimation methods under small samples for Structural Equation Models (SEMs). Based on this literature review, we conclude that with small samples the use of Bayesian methods with only default priors can lead to severely biased results. We end the chapter with recommendations for researchers on analysing a small sample size and on how to specify thoughtful prior distributions.
In Chapters 2 and 3, we discuss the results of simulation studies. Based on these studies we advise researchers with small samples to specify informative priors. We recommend researchers take the most careful approach possible: start with carefully constructing prior distributions; and assess the impact and robustness of the specified priors through an extensive sensitivity analysis. When researchers are not able or willing to include prior information, we advise to use the twostep method or factor regression score. These methods are a safer choice than maximum likelihood estimation, as those led to higher convergence rates without negative variances, more stable results across replications and less extreme parameter estimates than maximum likelihood estimation with small samples.
In the final chapter, we discuss in a non-technical tutorial the risks of using Bayesian estimation while blindly relying on built-in software default priors when samples are small. Also, we demonstrate an online educational Shiny app, in which users can play around with varying sample sizes and prior settings to investigate the impact of priors on the results. In addition, we provide guidelines on how to use Bayesian SEM in a thoughtful way when samples are small.
Supervisors
Prof. dr. A.G.J. van der Schoot
Prof. dr. L. Wijngaards-de Meij
Financed by
NWO – Vidi grant Dr. Rens van de Schoot
Period
1 January 2016 – 2 June 2023