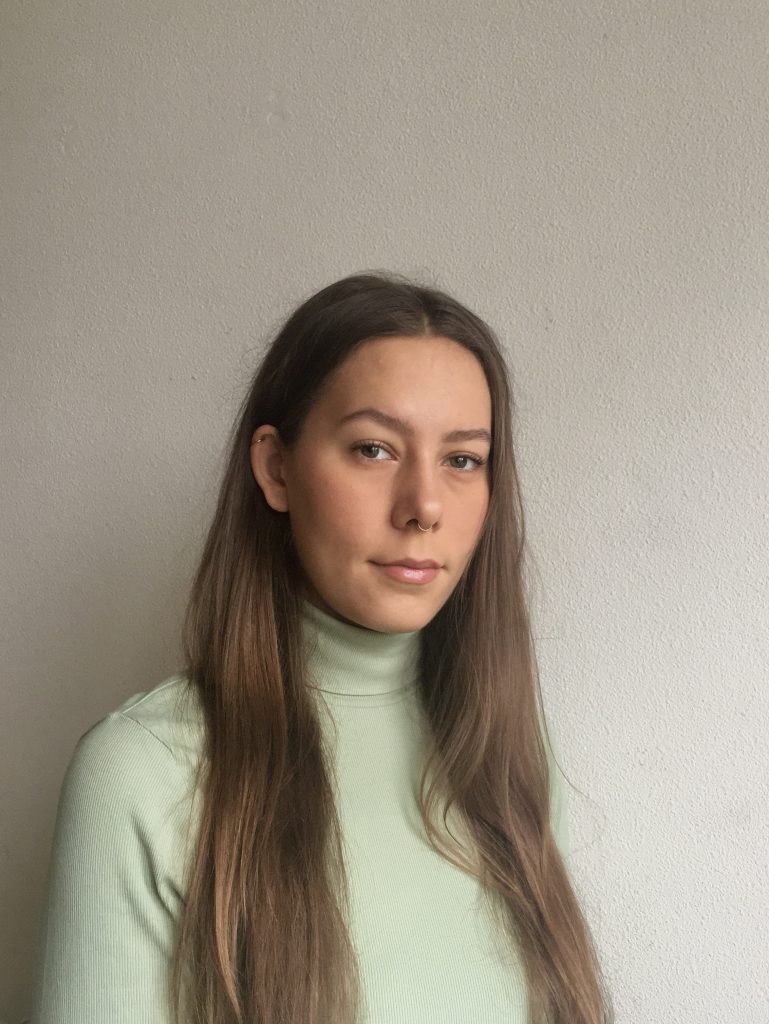
Social and Behavioural Sciences
Psychology – Clinical Psychology
Leiden University
E-Mail
Website
Project
Early Warning Signals of Depression Onset
Depression is common, costly, and debilitating (e.g. Kessler et al., 2005, Lopez et al., 2006), but treatment effectiveness remains disappointing (e.g. Cuijpers et al., 2018; van Zoonen et al., 2014). Experts agree that focusing on prevention is crucial to move forward (e.g. Cuijpers et al., 2013). Detecting who needs prevention when is the prerequisite to effective preventive interventions – yet reliably forecasting an individual’s risk for transitioning into depression in the near future is currently not possible.
This PhD focuses on the theory-driven personalized prediction of depression onset by incorporating concepts from clinical and complexity literature. The project will investigate whether early warning signals (EWS) in the relations between and severity of symptoms precede transitions into depression, whether such transitions are abrupt or gradual, and how this may differ between individuals. The results will inform the building of a personalized early warning system for depression onset, WARN-D.
To enhance the prediction accuracy of depression onset, this PhD project draws from complexity theory and the network approach to psychopathology (Borsboom, 2017; Cramer et al., 2010; Fried et al., 2017): Depression is conceptualized as a within-person system of problems influencing each other over time. We will use dynamical network models (e.g. Bringman et al., 2013) to study the within-person temporal dynamics of depressive symptoms and related biopsychosocial factors, combining subjective self-reports with more objective activity tracking data from wearables.
This PhD project aims to evaluate and compare different methods in the process:
Firstly, to differentiate stable fluctuations in the mood system from instability indicating transitions into depression, it is important to adapt a time-varying perspective. This PhD project will compare different emerging analysis approaches for doing so (see Bringman et al., 2021). Secondly, across different disciplines studying complex systems, a variety of EWS indicating that a system will transition from one stable state to another (e.g. from healthy to depressed) have been proposed, along with different perationalizations and methods for assessing them.
This PhD project will assess the utility of different EWS in the context of depression onset and compare the approaches to detect these EWS (such as critical slowing
down, e.g. van de Leemput et al., 2014).
Supervisors
Prof. dr. A.J. W. van der Does
Dr. E.I.F. Fried
Period
12.04.2021 – 11.04.2025