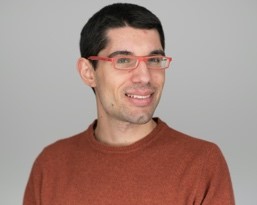
Methodology and Statistics
Tilburg University
Academic webpage: Eduardo Constantini
Project
Treatment of Missing Data in High-Dimensional Social and Behavioral Science Data
Properly handling missing data, by means of imputation of probable values, requires the specification of a model for the nonresponse. This process is key to achieve good performance of the imputation procedure and it is one of the most challenging steps of missing data handling.
The quality of any imputation model depends on how well it satisfies four main conditions. First, the distributional assumption made for the missing data must closely resemble its true missing data distribution (Schafer, 1997, p. 9). That is, the imputation model should reflect the distribution of the variable imputed. Second, all variables that are related to the nonresponse must be included in the imputation model, so that the assumption of Missing at Random is as plausible as possible (van Buuren, 2018, p. 168). Third, all important non-linearities and interactions between recorded variables should be included in the imputation model (Hippel, 2009). Finally, the imputation model should not be over-specified (i.e., containing too many parameters) (Graham, 2012).
The difficulty in satisfying these conditions is exacerbated in high-dimensional datasets, which are characterized by a number of columns (recorded variables, p) that is larger than the number of rows (observations, n). In such situations, the system of equations required to model the data becomes undetermined, meaning that there are fewer equations than parameters that have to be estimated. In an imputation context, this translates in overspecification of the imputation model and hence violation of the fourth condition. However, using fewer predictors would make meeting the second condition more difficult (i.e., including all important missingness predictors). Furthermore, trying to meet the third condition, by including interactions and non-linearities in the imputation model, might increase the dimensionality of an imputation problem to a p > n situation. This could cause an otherwise well-behaved estimation procedure to have singularity issues, such as not estimable model parameters.
High-dimensional imputation issues are well known to researchers working in many different disciplines. Examples are biologists working with gene datasets, social psychologists working with experience sampling and modest sample sizes, and sociologists working with longitudinal datasets.
In the social sciences, one of the areas that is most afflicted by missing imputation issues is longitudinal survey analysis, and their treatment is still challenging. Consider for example the
European Values Survey (EVS), a largescale cross-national longitudinal survey on human values in Europe, or the Longitudinal Internet Studies for the Social sciences (LISS) online household panel. When applied to such large datasets, some of the most popular missing data treatments, such as Multiple Imputation (Rubin, 1987; Little & Rubin, 2002) and Full Information Maximum Likelihood based approached (Anderson, 1957), are challenged by computational limitations of high-dimensional datasets. The large number of items recorded, together with the longitudinal nature of these surveys and the necessity of preserving complex interactions and non-linear relations, easily produces high-dimensional (p > n) imputation problems. A straightforward application of algorithms such as EM (e.g. Schafer, 1997) or Multiple Imputation by Chained Equation (MICE, van Buuren, 2018) is usually unfeasible in these conditions.
Consider for example the MICE algorithm. MICE specifies an implied multivariate distribution for the collected data, through a series of univariate full conditional distributions. Imputation is done by iteratively cycling over conditionally specified imputation models for each variable afflicted by missing values. At iteration t, an imputation model for each target variable ?? is trained using its observed part ??,??? as dependent variable, and the remaining data columns ?−? as predictors, with their possible missing value filled-in as in iteration t-1. With a longitudinal survey, the inclusion in any full conditional imputation model of all predictors, interaction and polynomial terms necessary to meet the assumption of MAR and to preserve the complexity of the data structure, may yield the computational obstacles of modeling high-dimensional data.
Many solutions have been proposed for dealing with missing values in high dimensional contexts, but these methods have been mostly aimed at improving the precision of the imputations (i.e., imputing values as close as possible to the true unobserved ones), rather than yielding valid inference (Rubin, 1976). The goal of our project is to design proper imputation procedures (Schafer 1997, p. 143) that yield statistically valid inference for quantities of interest (e.g., regression coefficients of an analysis model fitted to the imputed data) in high-dimensional datasets. In other words, we are interested in obtaining imputations that grant estimates (?̂), of the parameters (?) in some analysis model fitted to the treated data, that are unbiased and confidence valid (Rubin 1987, p.118-199; van Buuren, 2018, p. 41), not in minimizing the root means squared error of imputation.
We aim to reach this goal by integrating in multiple imputation algorithms, statistical modelling techniques coming from the high-dimensional prediction literature, such as frequentist regularized regression (e.g. Tibshirani, 1996), Bayesian lasso (Park & Casella, 2008), and Bayesian Additive Regression Trees (Chipman et al., 2010)). The potential for this approach is to obtain algorithms that meet as closely as possible the requirements for the correct specification of imputation models, and by doing so, produce proper imputations, in high-dimensional contexts.
Supervisors
Prof. dr. K. Sijtsma, dr. K.M. Lang, dr. T. Reeskens
Financed by
Tilburg University
Period
1 September 2019 – 1 September 2023