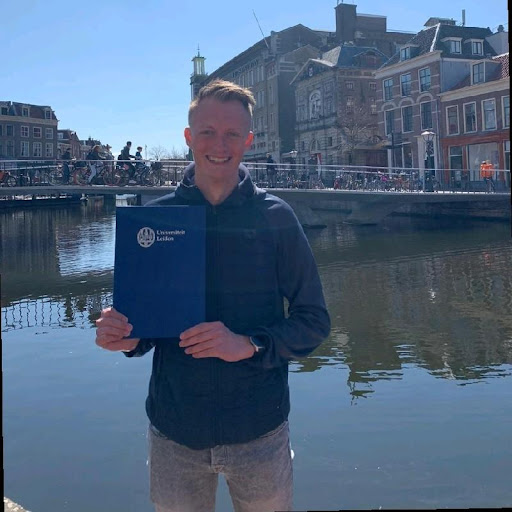
Project
Improving the Accuracy of Aggregate Statistics with Quantification Learning
The topic of the proposed research is quantification learning. Quantification learning aims to estimate a property of a population, such as the proportion of houses with solar panels in a community (Forman, 2005). The defining feature of quantification learning is that it does not only rely on classifications (for example, does a house have a solar panel or not) performed by humans but also on classifications from machine learning algorithms, which crucially are typically more error prone than human classifications. Quantification learning has broad applicability, such as, estimating the proportion of solar panels in a specified area (Curier et al., 2018), quantifying the sentiment towards a given entity (Gao and Sebastiani, 2015) and measuring the general opinion in election polls (Hopkins and King, 2010; Wiedemann, 2019).
Let us illustrate using the example concerning solar panels why using standard approaches for aggregating classifications from machine learning classifiers leads to problems. Suppose we have classifications (solar panel house vs no solar panel house) from a machine learning classifier for all houses in the Netherlands and we want to obtain the proportion of houses with a solar panel. Even when the classifier is very accurate, the estimate obtained by simply counting the number of houses classified as having a solar can be severely biased. Suppose that the classifier can predict objects fairly accurate: 98% of the houses with solar panels are classified correctly (sensitivity) and 92% of the houses without solar panels are classified correctly (specificity). In the target population of 10,000 houses there are 1000 houses with and 9000 without a solar panel. Thus, the true proportion of houses with a solar panel, i.e. prevalence, is 10%. The machine learning algorithm classifies 98% of the houses with a solar panel and 8% of the houses without a solar panel as houses with a solar panel. This aggregates to 1000 x 0.98 + 9000 x 0.08 = 1700 houses classified as a house with a solar panel installation. Thus, we estimate the proportion of houses with a solar panel as 17% instead of the true value of 10%: this is a relative difference of 70%. In statistical terms, we call this difference misclassification bias, and as the example demonstrates it can occur even when the classifier can predict every individual label with high accuracy (Scholtus and Delden, 2020; Schwarz, 1985).
Supervisors
Prof. dr. M.J. de Rooij
Dr. J.D. Karch
Dr. Q.A. Meertens MSc
Period
1 June 2021 – 1 June 2025