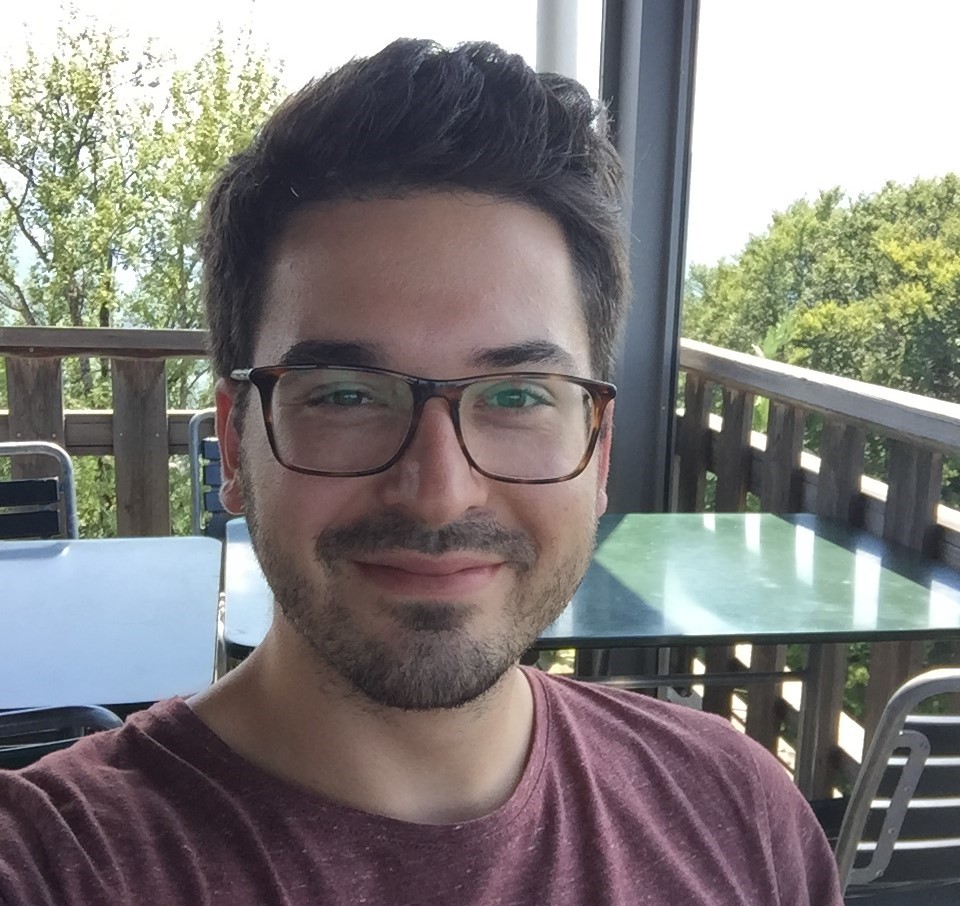
Psychometrics and Statistical Techniques
University of Groningen
Academic webpage Maximilian Linde
Project
Back to Bayesics: Solving the Reproducibility Crisis in Biomedicine
An alarmingly high proportion of biomedical research findings cannot be replicated or prove to be exaggerated, attesting to the presence of a reproducibility crisis in biomedicine. There is a multitude of sources for this reproducibility crisis. A major culprit is the inappropriate usage and evaluation of statistics (Benjamin et al., 2018; van Ravenzwaaij & Ioannidis, 2017). In particular, replication failures are partly due to flawed statistical techniques and the unavailability of accessible and more proper methods. The biomedical literature is cluttered with applications of frequentist statistics (Chavalarias, Wallach, Li, & Ioannidis, 2016), despite its disadvantages and pitfalls (Benjamin et al., 2018; Goodman, 1999a, 1999b; Wagenmakers, 2007). Two of the most important problems are the following: First, frequentist statistics does not allow for the quantification of evidence in favor of the null hypothesis (e.g., Wasserstein & Lazar, 2016). Second, researchers employing frequentist statistical methods are obliged to adhere to an a priori determined sampling plan (e.g., Rouder, 2014). That is, it is not legitimate to decide to stop or continue data collection based on inspection of interim results because this practice leads to inflated type I error rates (Armitage, McPherson, & Rowe, 1969).
Bayes factors (Jeffreys, 1961) express the relative likelihood of the data under the null and alternative hypotheses in the form of an odds ratio. For instance, if the Bayes factor is 15, the data are 15 times more likely to have occurred under the alternative hypothesis than under the null hypothesis. Conversely, a Bayes factor of 0.2 indicates that the data are 5 times (1/0.2) more likely under the null hypothesis than the alternative hypothesis. Therefore, it is possible to quantify evidence for both hypotheses in an intuitive way. Additionally, optional stopping and sequential testing bear no problems within the Bayesian framework (Rouder, 2014; Schönbrodt, Wagenmakers, Zehetleitner, & Perugini, 2017).
Although the basic theory of Bayesian statistics was developed centuries ago, it has been gaining traction within the scientific community for only a few decades (van de Schoot, Winter, Ryan, Zondervan-Zwijnenburg, & Depaoli, 2017). User-friendly software for Bayesian statistics is scarce, largely leaving Bayesian analyses to be a privilege for statistical experts. Fortunately, commencing efforts have been made to make Bayesian tools accessible for the wider audience. For instance, the ‘BayesFactor’ software (Morey & Rouder, 2018), written in R (R Core Team, 2019), enables researchers to calculate Bayes factors for various research designs. Available click-and-drop software include JASP (JASP Team, 2018) and Jamovi (The jamovi project, 2019). However, these tools are mainly developed for the social sciences. Many research designs that are common in biomedicine (e.g., superiority, equivalence, non-inferiority, survival, and relative risk designs) are not covered by these software packages. To rectify this problem, van Ravenzwaaij and colleagues (2019) developed the mathematical framework for the calculation of Bayes factors for superiority, equivalence, and non-inferiority designs. However, these tools still need to be implemented in a user-friendly software, so that a wide range of researchers can use them. Moreover, other common biomedical designs (e.g., survival analysis) need to be added to the collection of Bayesian tools implemented in the software.
The individual contents of this project will contribute to one overarching goal, which can be summarized under the following research question:
How can we properly quantify evidence for or against the efficacy of a treatment in biomedical research designs?
Biomedical data for superiority, equivalence, and non-inferiority trials are almost always analyzed within the frequentist framework, using null hypothesis significance testing and corresponding p-values (Chavalarias et al., 2016). To a large extent, this procedure is culpable for the prevailing reproducibility crisis in biomedicine. Due to the inherent limitations of the frequentist approach (Goodman, 1999a; van Ravenzwaaij et al., 2019; Wagenmakers, 2007), we propose that researchers use Bayesian statistical methods to analyze their data. Of course, to do this, researchers need proper and easy-to-use Bayesian tools. These tools will be developed and provided through this project.
Supervisors
Prof. dr. R.R. Meijer, dr. D. van Ravenzwaaij, dr. J.N. Tendeiro
Financed by
VIDI fellowship grant dr. Don van Ravenzwaaij
Period
1 September 2010 – 31 August 2023