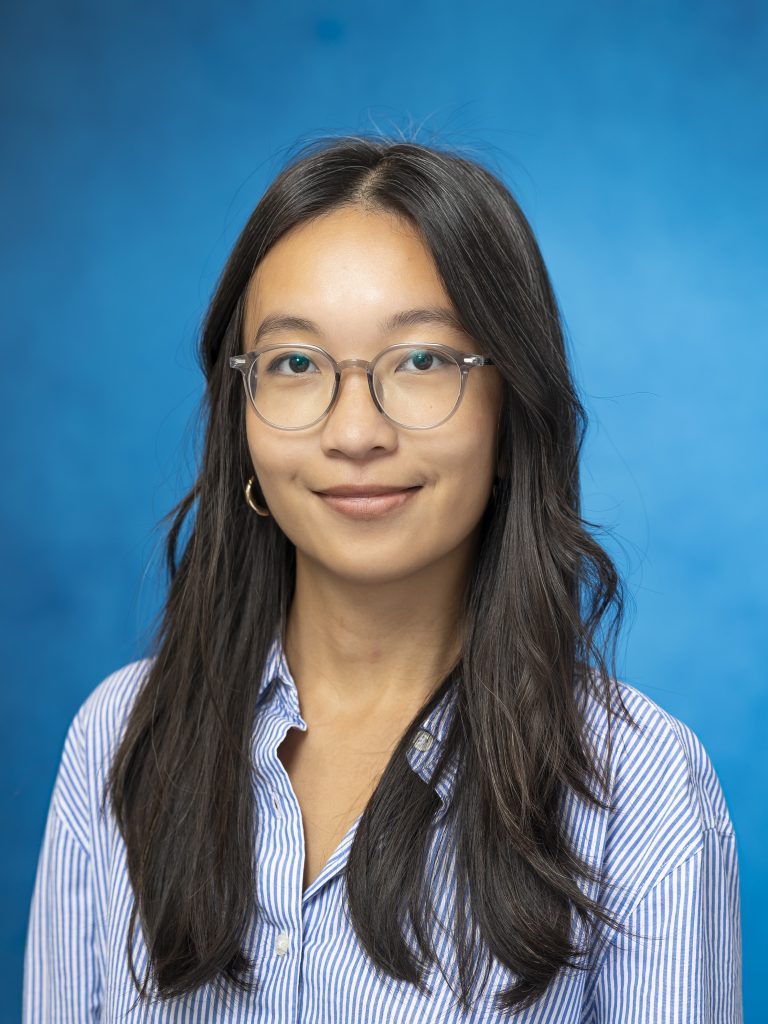
Methodology and Statistics
Social and Behavioral Sciences
Tilburg University
Email
Project
SEM 2.0: Towards personalized multi-disciplinary treatment plans
The next-generation approach to research is based on “big data” and complex models. On the one hand, the digital revolution has resulted both in technologically advanced measurement tools (e.g., wearable devices that track one’s physiology and location, genetic sequencing tools, or digital assessment tools used in educational testing) and an increase in available data; often, this leads to data with more variables than cases or so-called high-dimension low-sample-size (HDLSS) data. On the other hand research paradigms have shifted towards multi-disciplinary person-centered approaches. Behaviour and cognition are no longer studied from the psychological perspective only but also from other disciplinary perspectives such as the environmental, social, clinical, and biomolecular (see, e.g., van de Poll-Franse et al., 2022). Together with the acknowledgement that behaviour represents a complex interplay of multiple disciplinary domains comes the realization that a person-centered approach is needed (Bryan et al., 2021). Clinical and medical psychologists, for example, realize that there are large inter-individual differences in the treatment plans needed: for some patients poor outcomes mainly depend on behaviour (e.g., unhealthy lifestyle), for some on genetic constitution (e.g., immune deficiencies), and for some on a subtle interplay between genetic susceptibility and triggering/protective behaviour. Hence, explanatory models of the data are needed on which such person-centered approaches can build. As there is often limited prior knowledge available, this implies complex models characterized by relatively many unknown parameters compared to the number of cases.
Structural equation models (Kline, 2015) are particularly powerful tools for building explanatory models of behaviour and cognition: They model both the latent variables (with a so-called measurement model) and the relations between the latent variables (with a so-called structural model). However, currently available structural equation methods are not suitable for (large scale) multi-domain data (e.g., collected with the aim of developing personalized multi-disciplinary treatment plans). First, these methods do not scale up to the HDLSS setting: The parameter estimates are known to be unreliable when sample sizes are small and solutions may even not compute (in reasonable time); see Rosseel (2020). Second, they do not account for the multi-domain structure of the data: To obtain a good understanding of how behaviour and cognition depend on the multiple domains, domain-specific mechanisms should be disentangled from joint mechanisms. This implies constructing latent variables that account for the grouped or block structure of the variables (with each group/block referring to a particular domain). And last, currently available SEM methods do not support personalized approaches as this implies modelling (almost) at the level of the individual, which results in an extreme case of the HDLSS setting.
This project develops a regularized least-squares latent variable method, regularized LS-LV, that addresses the challenges posed by the modern research paradigms based on intensive collections of multi-disciplinary data and a desire to account for substantial heterogeneity in the underlying mechanisms. The method
(1) scales up with complexity such that it can be used when sample sizes are small, when the number of variables is high, and when differences among many (unknown) groups exist.
(2) yields insightful results by imposing simple structure not only within domain-specific data but also across domains to reveal the joint and domain-specific mechanisms that shape behaviour and cognition.
(3) takes a major leap towards person-centered modelling by building on regularization to avoid overfitting and on convex optimization and distributed computing to address computational efficiency.
Supervisors
Prof. dr. Katrijn Van Deun
Dr. Simone Oelermans
Period
1 September 2023 – 1 September 2027