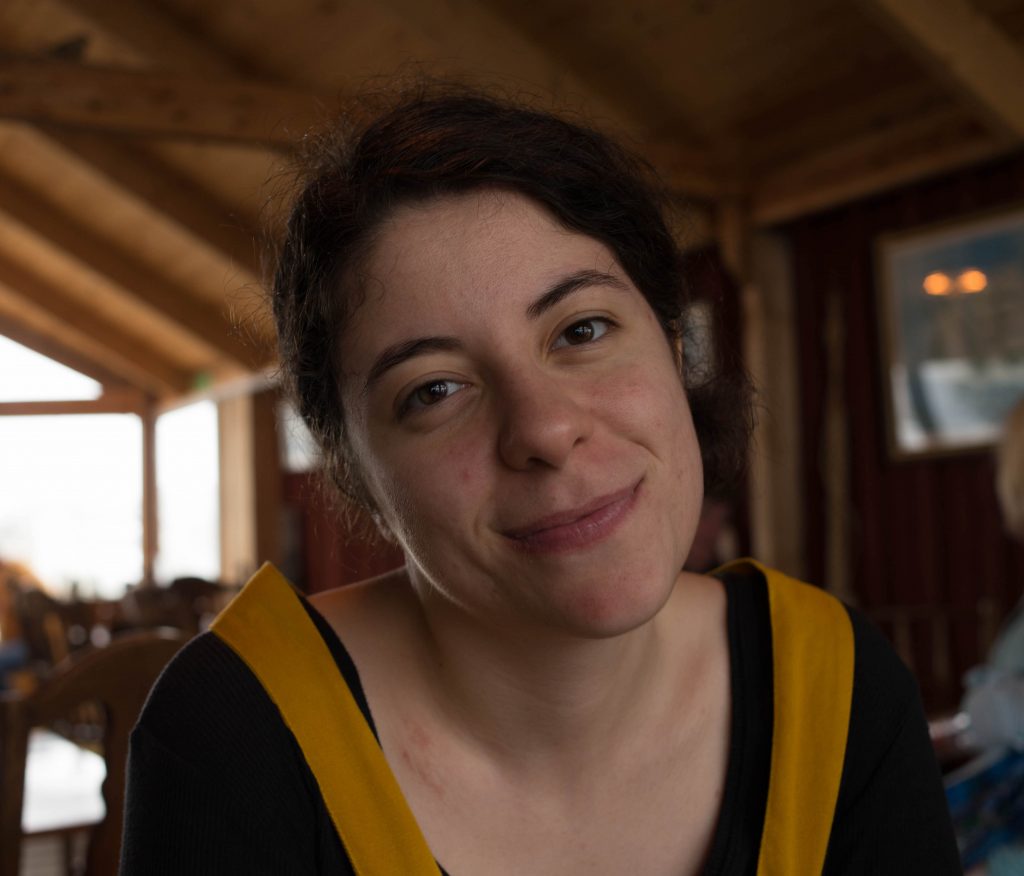
Social and Behavioral Sciences
Research Institute Child Development and Education
Child Development and Education
University of Amsterdam
Project
Dependent effect sizes in meta-analytical structural equation modeling (MASEM)
Meta-analytic structural equation modeling (MASEM) allows researchers to meta-analyze the relations between multiple variables in a given SEM model. Whereas traditional meta-analytic methods would need to carry out separate meta-analyses for each bivariate relationship, MASEM allows researchers to meta-analyze all the relationships simultaneously and thus the whole SEM model (Cheung, 2015). To this extent, another advantage of MASEM is that the included studies need not measure every variable of interest. This way, the analysis can take into account any study that includes at least two of the variables of interest and make the most of the available information in existing literature.
There are, however, some limitations associated with MASEM. Currently, the analysis only allows one effect size per bivariate relationship from each study, and the current practice when it comes to carrying out a MASEM analysis cannot properly deal with cases where there are multiple effect sizes available from the same study for the same relation. However, in practice, one study can report multiple correlation coefficients; there could be multiple informants, multiple time points, or multiple operationalization strategies. In fact, reviews of meta-analyses suggest that of the studies included in meta-analyses, a large percentage include dependent effect sizes (e.g. Rios et al., 2020). Existing applications either treat these effect sizes as independent, randomly select one effect size amongst many or create an average effect size. In their review article, Sheng et al. (2016) report that 22.7% of the studies with dependent effect sizes treat these as independent and 77.3% pick one effect size among many and disregard the rest. It is clear that with any of these strategies, there is either substantial information loss or the amount of information is overstated, which may lead to under or overestimated standard errors and results in biased estimates (Moeyaert et al., 2016; López-López et al., 2017).
In their article, Stolwijk et al. (2022) compared these three strategies, along with the univariate three-level modeling approach developed by Wilson et al. (2016) in an empirical application and found that different methods led to different results. These methods have not yet been compared across varying conditions in a simulation study, thus, within this PhD project, the initial stage will be to compare the existing strategies across a variety of conditions to assess their performance. After establishing how “harmful” these strategies can be, the next stages of the project is to then to merge the ideas from MASEM and multivariate multilevel modeling, and also look into extending the robust variance estimation (RVE) method to deal with dependent effect sizes (Hedges et al., 2010) to the MASEM context. The newly developed and extended methods will also be evaluated in simulation studies. This PhD project can be considered as an IOPS project since it involves both the comparison of existing methods, and also the development and extension of new methods.
Supervisors
Dr. S.J. Jak
Financed by
NWO – Vidi grant
Period
January 2022 – January 2026